Balancing explainable and responsible AI in insurance
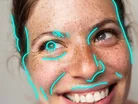
Artificial intelligence (AI) is transforming the insurance industry with the potential to improve underwriting, claims management, fraud detection, and customer service. However, as AI systems become more sophisticated and pervasive, concerns are growing about their transparency, accountability, and impact on society. Two concepts that are gaining importance in this context are Explainable AI (XAI) and Responsible AI (RAI).
What's the difference between explainable AI and responsible AI?
Explainable AI refers to the ability of AI systems to provide explanations for their decisions and actions in a way that humans can understand. XAI is important for ensuring transparency and accountability in AI decision-making, as well as for building trust between humans and machines. In the insurance industry, XAI can help insurers explain how they arrived at their underwriting or claims decisions, which can be particularly important in cases where the decisions may have significant financial or social impacts.
For example, suppose an AI system is used to determine the premiums for a life insurance policy. In that case, XAI can help the insurer explain how the system arrived at its decision, which factors were considered, and how the applicant's risk profile was assessed. This can help to address concerns about potential bias or discrimination in the decision-making process and ensure that the system is fair and equitable.
Responsible AI, on the other hand, refers to the practice of developing and deploying AI systems in a way that aligns with ethical and social values. RAI involves considering the potential impacts of AI systems on society as a whole, such as the displacement of workers or the perpetuation of bias and discrimination. In the insurance industry, RAI can help insurers ensure that their AI systems are designed to respect individual privacy, fairness, and human rights.
For example, suppose an AI system is used to detect fraudulent claims. In that case, RAI can help the insurer ensure that the system is not unfairly targeting certain groups of claimants or infringing on their privacy rights. RAI can also help to ensure that the system is not replacing human judgment entirely, as this could have negative impacts on the quality of the claims decision-making process.
What are the potential pitfalls of AI?
Responsible AI is particularly important in insurance, where decisions made by AI systems can have far-reaching impacts on individuals and society as a whole. For example, if an AI system perpetuates biases based on race, gender, or other factors, it can lead to unfair and discriminatory outcomes. Similarly, if an AI system collects and uses customer data in a way that violates their privacy or human rights, it can erode trust in the insurance industry and damage the reputation of insurers.
While both XAI and RAI are important for building trustworthy and ethical AI systems, there can be tensions between the two concepts. For example, in some cases, providing a detailed explanation for an AI decision may compromise the privacy or confidentiality of the data used to make the decision. In other cases, an AI system may be designed to make decisions based on complex algorithms that are difficult to explain in a way that humans can understand.
In order to ensure that AI systems are both explainable and responsible, insurers need to take a holistic approach to AI governance. This involves developing policies, procedures, and technical standards that promote transparency, fairness and accountability in AI decision-making. It also involves ensuring that AI systems are subject to rigorous testing, validation and ongoing monitoring to detect and mitigate potential risks and biases.
To achieve these goals, insurers can use a variety of tools and techniques, such as model explainability algorithms, fairness metrics, and human-in-the-loop processes. They can also work with external partners, such as academic researchers and civil society organisations, to ensure that their AI systems are aligned with ethical and social values.
In conclusion, explainable AI and responsible AI are two key concepts that are essential to building trustworthy and beneficial AI systems in insurance. By promoting transparency, fairness and accountability in AI decision-making, insurers can enhance trust, improve outcomes, and ultimately create value for both customers and society as a whole. To achieve these goals, insurers need to take a holistic approach to AI governance that balances the benefits and risks of AI and aligns with ethical and social values.
About the author
Janthana Kaenprakhamroy is Co-Founder and CEO of Tapoly, one of the first on-demand insurance providers for SMEs and freelancers in Europe. Kaenprakhamroy has been listed by Forbes in the Top 100 Women Founders to watch, and among the Top Ten Insurtech Female Influencers according to The Insurance Institute. She is a former chartered accountant and internal audit director at investment banks, having previously worked at UBS, Deutsche Bank, JPMorgan, and BNP Paribas.
Featured Articles
CRIF and Crawford integration cuts insurance claims processing time by 30% for UK businesses through bank account data access
Qover partners with premium car brands BMW and MINI to deliver embedded insurance solution with omnichannel capabilities for the Irish market
Marshmallow’s new funding supports aim to leverage proprietary underwriting technology beyond motor insurance while planning cross-border expansion