How can insurers realise the true value of AI?
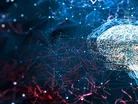
As artificial intelligence (AI) and digital transformation find their way into every aspect of our daily lives, we are gradually seeing changes taking place in different sectors.
Progressively, AI is permeating the insurance value chain and it is set to significantly transform the industry. In product development, AI is enabling insurers to create more profitable and effective products based on insights from past claims and product uptake in the market.
Underwriters are also using AI to assist in creating a better understanding of risk for new and underserved markets.
Also, with the integration of conversational interfaces, there are improvements to customer service and advances in fraud detection are enabling efficient claims processing.
Yet, despite these AI transformations, the potential of AI has yet to be fully realised. For now, AI’s role in the insurance industry is largely limited to optimising existing business processes rather than developing new and disruptive business models.
There are a few key reasons for this:
AI is highly centralised
Insurance companies are currently integrating applications to use machine learning/deep learning (ML/DL) models to gather insights from the enormous amount of data they generate.
However, the increasing complexity of ML/DL models requires enormous amounts of compute power. According to research from OpenAI, the computing power required over the past few years has increased 300,000 times between 2012 and 2018.
At the moment, only a few niche technology companies have the skilled data scientists required to develop complex models, enormous datasets required to train these models, infrastructures that are required to deploy these models at scale.
Lack of AI ready data
The problem is not the lack of data in the industry, but that insurance entities are struggling with an enormous amount of data that isn’t AI-ready.
Data needs to be cleaned, integrated, moved to appropriate infrastructure, governed and managed continuously.
It also has to be labelled correctly for accurate decision making; this labelling process is time-consuming and expensive. Further, negative data is not easily available to train ML/DL models in failure scenarios.
For example, you would never send a fleet of self-driving cars out on a mission to crash on purpose just to help an AI decide what went wrong at a crash scene.
Still evolving explainability of AI decisions
For industries such as insurance that operate in strict regulatory environments, the opaqueness of these models is an issue. Models can be created with an unintentional bias that skews decisions in unexpected ways.
The technology to explain why and how AI models made their decisions is still in its initial stages.
Overcoming AI obstacles
The good news is, these obstacles are temporary and should not be seen as deal-breakers.
There are ways to overcome them. For example, tech companies are pursuing multiple strategies to increase AI’s value and boost its adoption.
Some solutions involve combining emerging technologies while others involve creating incentives to contribute new information or filter data that feeds AI applications.
Either way, the broader concept is the same — for insurers to move AI away from large tech companies that solely manage solutions and put it into a decentralised, democratised form. Here’s how:
- Differentiating AI models
Access to unique data means insurers will be able to find ways to train their ML/DL models to help distinguish their business. This will allow insurers to capitalise on AI and differentiate themselves from other insurers that are using AI for similar use cases.
- Developing scaled-down algorithms
Many algorithms require large, expensive datasets to identify patterns and generate insights. Developing ML/DL algorithms that can function on comparatively small datasets will make them less expensive and more widely available.
- Create an AI ecosystem
As AI decentralises, insurers will find benefits in creating an ecosystem in which datasets and models can be shared among partners and even other insurers.
For example, an individual insurer would typically build its own datasets to train ML/DL models to process handwritten notes by adjusters, estimation documents, images and videos, and the plethora of information online about the insured.
They could use their own datasets in addition to those from the ecosystem to create new value-added features in the ML/DL model.
Realising true potential of AI
Insurers are already reaping the rewards of AI and there is an understanding that this technology has the potential to create much more value in the future.
However, the way to achieve that has been less clear. Taking a decentralised approach will provide data management with the necessary resources and training needed to enable the technology to grow.
Improving model making, sharing datasets and encouraging contributions from policyholders will make this fast-evolving technology truly useful and value-added.