Machine Learning boosts weather predictability for insurers
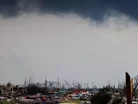
Machine Learning (ML) technology is making predictions in crisis weather events more accurate, helping insurers create increasingly effective and financially sound reinsurance design, say experts.
The promising data, which was gleaned using a convolutional neural network (CNN), was released at the 2021 Actuaries Summit in Australia. This year’s virtual event runs from April 27th to May 21st.
Extreme weather and insurance risk assessment
Statistical reports show that 2020 suffered a dramatic year in terms of climate and weather induced crisis compared to previous years. Although natural disasters in Europe remained low, the US, China and the North Atlantic saw extreme weather and damage.
- A record hurricane season: More storms in the North Atlantic than ever before
- Historic wildfires in the western US
- Worldwide, natural disasters produced losses of US$ 210bn, with insured losses of $82bn
- Floods in China were responsible for the highest individual loss of $17bn, only around 2% of which was insured
- Global losses from natural disasters in 2020 amounted to $210bn
Currently, the ability to predict extreme weather events and cycles such as the El Niño-Southern Oscillation (ENSO), Indian Ocean Dipole (IOD) and Southern Annular Mode (SAM), are limited from six to 12 months.
The new modelling techniques, say experts, will be critical in lengthening the forecasting range as insurers rely on technology and predictive analytics to assess the crisis risk.
The current limitation has resulted in disclosure requirements “leap-frogging” the capabilities of climate science by at least a decade, say experts, who noted that this natural variability is a key element of uncertainty in climate risk modelling.
Extending the forecasting ability by an additional six months will be vital in helping insurers manage pricing, reinsurance design and budgeting and experts are hopeful technology may hold the solution.
Machine Learning in risk management insurance
Suncorp Natural Perils Senior Pricing Advisor Tatiana Potemina, explained, “Improvements so far have been incremental rather than a step change but machine learning and neural networks are increasingly being used and the results are really promising.
Potemina said the new techniques can predict climate fluctuations up to 17 months in advance, and called the new development a significant step in crisis and risk modelling.
“There are some promising results there. For insurers, it’s important to understand natural climate variability because without this understanding some incorrect assumptions can be made about the trend.”
A new scientific paper entitled Deep learning for multi-year ENSO forecasts, shows that although the current system of modelling produces strong predictions, it’s only offered lead times of up to 12 months. Long-lead forecasts would be valuable for managing policy responses.
According to reports, the new study shows that a statistical forecast model employing a deep-learning approach produces skilful ENSO forecasts for lead times of up to one-and-a-half years.
Potemina said the study utilised ‘transfer learning’ to train a CNN on historical simulations and reanalysis. The research revealed the correlation skill of the CNN model was “much higher than those of current state-of-the-art dynamical forecast systems”.
She continued, “The CNN model is also better at predicting the detailed zonal distribution of sea surface temperatures, overcoming a weakness of dynamical forecast models,” the paper says.
“The CNN model is a powerful tool for both the prediction of ENSO events and for the analysis of their associated complex mechanisms,” Potemina added.
Featured Articles
Monzo targets underinsured market with flexible policy as research reveals half of UK renters lack coverage
Indian IT firm Zensar to modernise infrastructure as insurer separates from Tesco Bank following Barclays acquisition
BRC survey shows two-thirds of retailers plan price hikes due to national insurance increases, with food inflation potentially reaching 4.2%