Machine learning and deep learning in the insurance space
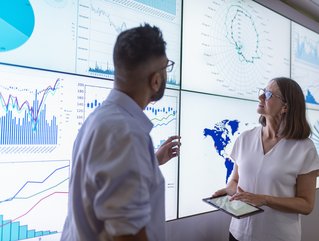
Machine learning and deep learning have really taken root in the insurance sector over the last couple of decades, building on the foundational layer set by artificial intelligence (AI) technologies to better contextualise data and create data-led outcomes.
The result is a gradual automation of the insurance value chain, helping to remove manual effort from previously arduous and cumbersome tasks – and helping insurers to realise tangible gains across risk, claims speed, and fraud prevention.
Indeed, machine learning and deep learning are such promising technologies that McKinsey estimates that the whole sphere of artificial intelligence will drive value within the insurance industry of up to US$1.1tn a year.
What’s the difference between ML and DL?
Before we can fully understand the advantages that machine learning (ML) and deep learning (DL) can bring to the insurance industry, it helps to be fully cognisant of the differences between the two. Both begin with artificial intelligence (AI) – which is obviously the ability for computers to process and contextualise data in the same way a human could.
Machine learning utilises AI to make data-driven predictions based on the data and learned experiences. In contrast, deep learning is an additional layer of ML where algorithms attempt to model high-level abstractions in data.
One use-case that already exists for deep learning is in improving the accuracy of risk forecasting, particularly in insurance lines where there is a lot of structured and unstructured data together – like auto insurance, for example.
LexisNexis Risk Solutions surveyed more than 300 insurance professionals in the fields of data science, analytics, actuarial, technology, underwriting, product management and claims working for some of the 100 leading insurance carriers in the US.
The findings from that poll show that three-quarters of insurance-industry respondents think AI and ML can provide a competitive advantage to carriers, while more than six in 10 respondents (62%) work at companies that have already started to adopt AI and ML.
LexisNexis Risk Solutions say that ML and DL can help insurers make faster, more informed decisions; maximise growth and profitability across the entire value chain; and adapt better to disruption and external forces.
Just the beginning for AI and ML in insurance
“Carriers have only started to realise the benefits of using AI and ML across their organisations – especially in marketing, underwriting and claims,” says John Beal, Senior Vice President for Data Science at LexisNexis Risk Solutions. “AI and ML applications in the claims area, in particular, can provide carriers with an opportunity for quick wins through faster claims settlement and improved fraud detection. In the long-term, carriers that are best able to hire the right talent to operationalise AI and ML applications and apply the learnings to their business can unleash the full benefits of their efforts.
“Carriers are generally positive about their use of AI and ML and its value in helping them achieve a competitive advantage. Our study also looked at the specific challenges associated with AI and ML implementations. Financial concerns – such as the cost of implementation, uncertainty around ROI and competing priorities – were cited by 93% of adopters. In addition, three major areas pose the biggest issues for carriers: staffing, data, and compliance and regulatory challenges.”
Still a role for humans in an ML/DL world
Despite a rapid evolution in the underlying technology, and inevitable optimism about what ML and DL can bring to the insurance sector, there are still potential pitfalls and limitations. One oft-discussed topic of controversy is the effect on the future labour market, but “it is implausible that AI will replace humans entirely,” says Oliver Tearle, Head of Innovation Technology at The ai Corporation (ai).
“The role of a human – interpreting, analysing, understanding, and compensating for data and events outside of the realms of the model – is still central to many processes. As a result, there is a role for humans and machines in any business process, and instead of man versus machine, the approach should be man and machine.”
Tearle continues: “AI models live or die by the data they are trained on. Up to 80% of the time it takes to develop an AI model is devoted to ensuring the data is of a high standard and packed with helpful information. Often, smaller and more information-dense data sets perform better than larger, untreated ones. AI is still bound by the limitations of the data, as well as the 'ground truths' it's fed in a classification modelling scenario.”
As an example, he says that “a typical fraud model will only utilise what is known and confirmed to be fraud, as this ensures the model can detect that fraud correctly in the future”. Tearle recommends that ML-driven strategies are routinely checked and overseen by humans to ensure the integrity of the decisions.
Generally speaking, the hurdles to effective ML and DL adoption are many and complex: they range from the cost of adoption and the sourcing of talent to potential regulatory implications and data challenges (such as ensuring the quality of data, normalising data across sources, and handling the need for increased volume of data).
“AI and ML are more than just buzzwords in the insurance industry,” John Beal continues. “These technologies can help carriers streamline their processes, better meet customer expectations and get the most out of their talent. Insurance carriers that have adopted AI and ML are already seeing benefits, including more efficient processes, better customer targeting and improved fraud detection.
“Most respondents agree that AI and ML will offer carriers a competitive advantage, define industry leaders, and are being used and invested in today. In addition, the majority of respondents recognise that the adoption of AI and ML applications is imperative for business success and that will continue to rapidly grow in importance over the next three years. However, respondents also said that it is important to be able to explain how AI and ML will be used in insurance decisions, otherwise AI and ML applications may be blocked or limited by regulatory and legal bodies.
“Respondents have four main areas of concern around AI and ML—financial, staffing, data and compliance challenges. While none of these should prevent a carrier from starting or advancing their AI and ML initiatives, carriers should be cognizant of the nuanced challenges within each area and seek a partner that can help optimise the value of their AI and ML investments.”